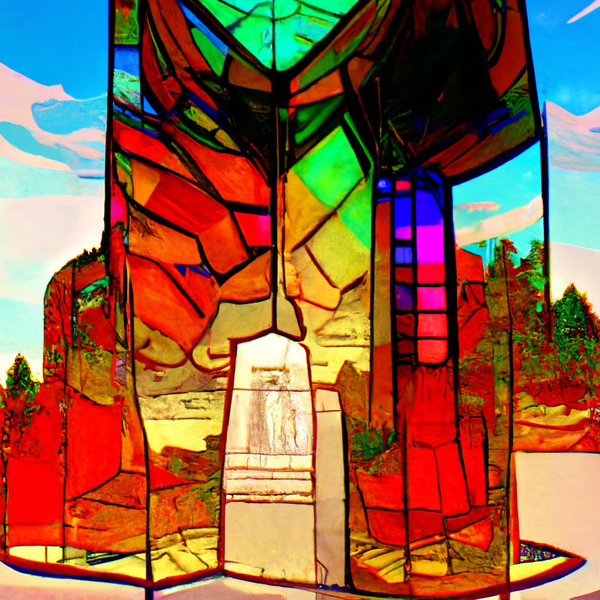
Institution Size as a Window into Cultural Evolution
September 20, 2023
Originally published at Economics from the Top Down
Blair Fix
One of the challenges of studying the past is that time has a maddening way of erasing evidence. Take the origin of life — the ultimate cold case. Had the first organisms been wise enough to fossilize themselves, we would know exactly how life began.
Fortunately, the first organisms did preserve themselves, at least in rough form. That’s because life is itself a kind of time capsule. As life evolves, it keeps a record of the past — a record written in the size distribution of organisms. Bacteria were the first form of life, and today they remain the most numerous organism. More recent inventions add to life’s complexity, but never erase its simple beginning. The result is that the size composition of life, as it exists today, is a reminder of Earth’s deep past.
I think something similar is true of human culture.
In this essay, I explore the idea that the size composition of human institutions, as it exists today, provides a record of cultural evolution. The idea sounds crazy at first. But the more I look at the evidence, the more plausible the idea seems. There are startling similarities between the niche structure of US institutions, and the niche structure of the rest of life on Earth. And that makes me wonder … is cultural evolution replaying (in accelerated form) the same game that life has played for billions of years? It’s too early to say for sure. But the evidence is tantalizing. Let’s have a look.
The past is always smaller
To frame our evolutionary journey, we’ll start at the beginning … the very beginning. The universe was born about 13.7 billion years ago in a Big Bang that created everything we see today. Or more precisely, the Big Bang created the building blocks of everything that exists.
By being born hot and small, the universe did us a favor. It gave history a direction. We humans take for granted the fact that big objects are composed of small objects. Galaxies are made of stars, stars are made of atoms, atoms are made of nuclei, and so on. We (or at least we scientists) also take for granted the fact that this structure evolved from the bottom up, small to large.
Why is the universe built this way?
Physicist Eric Chaisson has spent his career thinking about this question. In his revelatory book Cosmic Evolution, Chaisson recounts the evolution of structure in the universe.1 History has a direction, Chaisson argues, because the universe is expanding. The importance of expansion stems from basic thermodynamics. What drives the growth of structure is the (net) flow of energy. And it’s the universe’s expansion that enabled this flow. (I’ll describe how it works in a moment.)
To convince yourself that energy flows are key for creating structure, try the following experiment:
- Fill a pot with water;
- Set the pot on the table;
- Wait for structure to emerge.
How long would you have to wait before ‘cells’ emerge, as shown in Figure 1? No one knows the answer for sure. But the heat death of the universe would probably come sooner.

Now let’s redo the experiment, but this time add a flow of energy:
- Fill a pot with water;
- Set the pot on a hot stove;
- Wait for structure to emerge.
Once the water is on the stove, how long will it take for ‘cells’ to emerge? Probably a few minutes. The reason stems from thermodynamics. When liquids are heated from below, they form convection cells that help dissipate energy. Although convection cells are a rather boring form of structure, the same principle applies to everything in the universe. Energy flow gives rise to complex structure.
Back to cosmic evolution. If the universe is our ‘pot’, where is its ‘stove’? Interestingly, the ‘stove’ is actually the universe’s expansion. The key thing about expansion is that it let the universe cool.2 And that let the fundamental forces work their magic. The result is that matter clumped together, ignited itself into stars, and eventually provided the ‘stove’ of life. Figure 2 shows a stylized picture of this process.

At this point, you might be wondering why I am talking about the universe’s expansion in an essay that is ostensibly about cultural evolution. Well, the point is that if the universe was not expanding, there wouldn’t be any culture to evolve. Moreover, it is because the universe is expanding that history has a direction. The past is always smaller.
Life, from small to big
Like everything else in the universe, life began small. It started out as simple cells, which gradually evolved into the big organisms that exist today. Figure 3 shows a stylized picture of the process.

Interestingly, although life got bigger over time, it never abandoned its previous niches. Today, the first form of life — bacteria — remains the most numerous. In fact, when we look at the size spectrum of life as it exists today, we implicitly peer into the history of evolution.
To that end, Figure 4 shows the size distribution of life. You can see that it clumps neatly into size niches, and that when we look at ever-larger organisms, we repeat life’s evolution. Life began as bacteria, which evolved into protists, which evolved into multicellular invertebrates, which became fish, which became mammals.

In ‘The Evolution of Big’, I reflected on these size transitions, and tried to situate human societies within the rest of life on Earth. As individual organisms, humans are unexceptional. (We’re the black dots in Figure 4.) But when we look at human groups, things get interesting.
Suppose we treat modern firms and governments as ‘superorganisms’. When we do that, we find that these ‘superorganisms’ extend the size spectrum of life by four orders of magnitude. Figure 5 shows the trend.

Looking at the evidence, it’s clear that the history of evolution is imprinted in the size spectrum of life. And we can thank the Big Bang for that. Because the universe (and everything within it) was born small, life had no choice but to erect itself from the bottom up.
Life’s major transitions
When it comes to the big picture of evolution, what stands out is not the incremental change, but rather, the epic-making transitions. The reasons for these transitions are complex in practice, but simple in principle. Transitions occur when life runs into design barriers.
Take bacteria as an example. Their simple design works superbly on the microscopic scale. But it would be disastrous if scaled to macroscopic proportions. And that’s why there are no bacteria the size of blue whales.
Although most of life’s major transitions occurred millions (if not billions) of years ago, they remain imprinted on modern life. To see them, we need only peer at life’s size niches. Figure 6 tells the story.

Behold human superorganisms at the top of the scale — the pinnacle of life’s size evolution. How did we get there? The answer is obviously that humans evolved. But more than that, our culture evolved.
Size niches of human institutions
With cultural evolution in mind, let’s zoom in on human ‘superorganisms’. Like the rest of life on Earth, it turns out that human institutions have size niches. Figure 7 shows the pattern for the modern United States.

With this evidence in mind, here’s a question (that is bound to be controversial): as we move up the size spectrum of US institutions, do we replay the history of cultural evolution? I think the idea is surprisingly plausible.
Small-group origins
At the bottom of the US institution-size spectrum we find private firms. Surely these groups are not humanity’s original form of organization?
No, they are not … just like modern bacteria are not the original form of life. Today’s bacteria have had billions of years to improve their design, so they can do things that early life could not. The same goes for ‘firms’. Today’s private firms are geared towards monetary exchange and organized around private ownership — things that ancient human groups did not do. Still, I think that small firms have much in common with ancient human groups.
The key is that small firms are, well, small. It’s on the small scale that human culture began. And I suspect that if we studied the day-to-day relationships within small firms, they would probably look similar to the relationships found in early human groups.
The origin of government?
Continuing up the size scale, the next institution to appear after ‘private firms’ is ‘local government’, which emerges when groups get close to about 100 members (Figure 8).

This group size is interesting because it’s close to ‘Dunbar’s number’. In the late 1980s, the anthropologist Robin Dunbar discovered a tight relation between the (relative) size of primate brains and the size of their groups. The larger the brain, the larger the group. When Dunbar extrapolated the trend to humans, he predicted a group size of about 150 members. (Incorporating uncertainty widened the prediction to between 100–230 members.)
Dunbar proposed that a group-size of 150 represented our ancestral state. It is the maximum size of group that we can form spontaneously, without formal organization. So it is fascinating that in the modern US, local governments start to appear around this size.
Also interesting is the fact that it is local governments that appear first. Does this tell us about the evolution of the ‘state’? We know that city states were the first form of entrenched government. So it is intriguing that thousands of years later, it is local governments that remain the first to emerge as we move up the spectrum of size.
The origin of capitalism?
Continuing up the size spectrum, next we get ‘public firms’ — companies traded on the stock market. In the modern US, these firms become prominent when groups exceed about 1000 members.

Here’s another controversial question: does the emergence of public firms tell us about the origin of capitalism?
The answer depends on how we define ‘capitalism’. Marxists like Robert Brenner think capitalism is defined by the spread of wage labor. World-system theorists like Immanuel Wallerstein and Andre Gunder Frank think the spread of international trade is most important. And more recently, Jonathan Nitzan and Shimshon Bichler have focused on the spread of ‘capitalization’ — the ritual of converting property rights into money.
Let’s suppose that Nitzan and Bichler are right, and that capitalization is the defining feature of modern capitalism. It follows that the origins of capitalism are tied to the evolution of the public firm. That’s because public firms take the ritual of capitalization to its most extreme. (We know the capitalized value of public firms down to the second.)
Today’s public corporations have their origins in the ‘joint-stock’ companies of the past — companies that have a surprisingly long history. (Historian Joe Carlen traces them back to 7th century China.) I won’t delve into this history here. But I think it would be fascinating to study the origins of capitalism in terms of the size evolution of joint-stock companies.
The origin of the modern state?
As we reach the size pinnacle of US institutions, we find state governments, followed by the behemoth federal government. It’s not a surprising result, but one that is still interesting.

In the social sciences, there is a bitter divide between people who study ‘firms’ (economists) and people who study the ‘state’ (political scientists). Economists look at firms and see ‘free markets’. Political scientists look at the ‘state’ and see the exercise of power. The two approaches seem completely at odds.
I think that studying the size spectrum of institutions can help remove this divide. The key is that the ‘free market’ of economic theory lives at the bottom of the institution-size spectrum among small private firms. As we move to larger institutions, there is less and less ‘free market’ to be had, even if the groups still call themselves ‘firms’.
No one would mistake Walmart’s massive chain of command for a ‘free market’. Instead, Walmart’s hierarchy has more in common with government bureaucracy than it does with the ‘perfect competition’ of economic textbooks. I think this is true as a general rule. As we move to larger institutions, they become more ‘state-like’. The official ‘state’ is simply the biggest brute of them all.
Interestingly, the blurred line between ‘firm’ and ‘state’ is evident in the history of the joint-stock company. When Europe conquered the world (starting in the 15th century), joint-stock companies were a key part of imperial expansion. Companies like the Dutch East India Company were ostensibly ‘firms’. Yet in the territories they occupied, these companies operated more like ‘governments’. For that reason, historian Arthur Weststeijn calls these behemoths ‘company states’.
What I like about this view of the state is that it allows for both continuity and disjuncture. As we move up the spectrum of size, groups become increasingly ‘state-like’. And yet the official ‘state’ has characteristics that are quite different from other groups.
Creeping bigness
Looking at life’s evolution, the biologist Stephen Jay Gould famously saw stasis rather than change. He had a good point.
If there was a universal evolutionary drive towards bigger size and greater complexity, the Earth would look wildly different than it does. The soil would not teem with bacteria, nor would the ocean be filled with phytoplankton. These tiny organisms would have long ago abandoned their niches, either by growing larger or by going extinct. Today, big life would be all that remains.
The fact that we don’t live on a big-organism-only Earth tells us something about evolution. According to Gould, it means there is no universal drive towards complexity. Instead, there is a ‘random walk’:
The vaunted progress of life is really random motion away from simple beginnings, not directed impetus toward inherently advantageous complexity.
In the ‘random-walk’ model, simple beginnings never go away. Instead, small organisms persist as the basis of life, on top of which a few organisms grow larger and more complex. Here’s Gould’s illustration of the creep towards complexity.

If Gould is correct, then life looks the way it does precisely because there is no universal drive towards complexity. Instead, there is a ‘random walk’ in all directions. Some organisms evolve to be bigger, others to be smaller. The catch, Gould observes, is that in the small direction, there is a ‘left wall of minimal complexity’ beyond which nothing can go. In other words, bacteria are about as small as life can get. (Viruses are smaller, but cannot replicate on their own.) But on the right side, there was no such wall. And so life took a random walk towards complexity.
The result is that life remains pressed against the lower size limit, while at the same time creeping towards bigness. This size creep, however, was not continuous. Instead, it was marked by long periods of relative stasis, punctuated with rare (but monumental) transitions. After 4 billion years of evolution, the outcome looks like Figure 12. Life is organized into different size niches, each of which solves specific design problems. And at the bottom of the size spectrum, life remains pressed against the lower wall.

Remarkably, a similar pattern emerges among human institutions, as shown in Figure 13. In the modern United States, private firms remain pressed against the lower size limit of 1 person. On top of this minimum social complexity live larger institutions, each occupying a specific size niche. As with organism niches, the existence of institution niches suggests an evolutionary history of what Stephen Jay Gould calls ‘punctuated equilibrium’ — long periods of stasis marked by brief moments of monumental transition. (We’re probably living through a ‘punctuation’ right now.)

What is fascinating is that evolution seems to be repeating itself. In effect, what happened to organisms is now happening to human institutions. But because we are now dealing with cultural evolution, the rate of change is orders of magnitude faster.
And that raises the question: what will the niche structure of human institutions look like in 1000 years? Will it have crept toward even larger scales of organization? Or will our ‘superorganism’ experiment have ended in disaster, marked by a dramatic collapse towards the small scale?
Only time will tell.
Sources and methods
Organism size and abundance
Data for organism abundance by size comes from Hatton et al., ‘Linking scaling laws across eukaryotes’. The caveat is that their dataset is not intended to be a complete and balanced accounting of life’s biomass. (Hatton simply uses what data is available). Therefore, my analysis of organism composition by size should be treated with uncertainty. That said, the various size niches of life seem so distinct that better data would likely not significantly change the results.
US institution size and abundance
US institution size data comes from a variety of sources, detailed below. It is a mix of empirical data, and simulated data derived from empirical trends. The data covers the years 1977 to 2006. Distributions shown in this paper plot the aggregate histogram over all years, with data in each year given equal weight.
Local governments
Local governments consist of counties, municipalities, townships, independent school districts, and ‘special districts’. Putting together employment data for all US local governments requires some work, as much of the data is not available directly.
The ‘Annual Survey of State and Local Government Finances’ reports employment for city governments in the historical file City_Govt_Emp
. For all other types of local governments, it appears that only financial data is available. (If you know where I can get employment data, please let me know.)
To estimate employment in these other local governments, I use financial data. I start by taking the city government data and regressing employment onto direct expenditures. This gives a function that predicts (in each year) employment from expenditures. I then use this function, together with the expenditure data from other local governments, to estimate their employment.
Adding up all these estimates accounts for about 70% of local governmental employment, as reported in the aggregate historical file Public_Emp
. To adjust for this undercounting, I take the local government histogram and increase the bin counts (uniformly across all bins) so that the counts sum to the aggregate employment values in each year.
State governments
Employment data is from the US Census, ‘Annual Survey of State and Local Government Finances’, historical data file Public_Emp
.
Federal government
Total federal employment is the sum of federal civilian employment (FRED series CES9091000001
) and military employment (FRED series B4280C0A173NBEA
).
Firm data
The firm-size data used here is partially empirical and partially simulated. Let’s walk through the steps of estimating the size distribution of US public and private firms.
The Business Dynamics Statistics (BDS) database from the US Census offers (to my knowledge) the most comprehensive accounting of the size distribution of US firms. The table bds_f_sz_release
reports binned size counts dating back to 1977.
The problem with the BDS data is that it is not helpful for understanding the size distribution of the largest firms. The BDS caps its bins at firms with 10,000+ employees. So we know how many firms have 10,000+ employees, but we don’t know how they are distributed. In contrast, I want firm size bins that go up to 1,000,000+ employees. I also want to differentiate between public and private firms — something that the BDS does not do.
My solution is to estimate public and private firm size distributions using a combination of the BDS data and the Compustat database. The Compustat database reports employment data for US public corporations. I use Compustat as my baseline data for constructing the size distribution of firms with 10,000+ employees.
Estimating the public-private firm mix
My first step is to estimate the public-private composition of large firms. To my knowledge, there is no database that exhaustively catalogues the size of all large US private firms. The closest such data I can find is the Forbes list of America’s Largest Private Companies. The list ranks private companies by revenue, but it also reports employment data. From the Forbes 2020 list, I take employment data for every private firm with 10,000+ employees.
(Side note: Forbes seems to keep older data under lock and key. If you know a free way to access older Forbes lists, please leave a comment.)
The next step is to use the Forbes and Compustat data to estimate how the public portion of firms varies by firm size. Looking only at firms with 10,000+ employees, I put firms into log-spaced bins by employment, and then count the number, N , of public and private firms in each bin. Within each bin, I then calculate the portion of firms that are public:
I find that the public portion of firms tends to increase with firm size, as shown in Figure 14.

My next step is to take the empirical trend shown in Figure 14 and fit it with a regression (grey line). I model the public portion of firms as a logarithmic function of firm employment, E :
Simulating the size distribution of public and private firms
With our regression for the portion of public firms, P_{\text{public}} , we are ready to simulate the size distribution of US public and private firms. The steps are as follows:
- Start with the Compustat database. In each year, put firms into log-spaced bins. Count the number of firms in each bin.
- For bins with 10,000+ employees, use the regression from Figure 14 to estimate the portion of firms that are public, P_{\text{public}} . We do this by evaluating P_{\text{public}} at the bin’s geometric midpoint.
- Estimate total firm counts (including private firms) within each bin. For each bin, take the Compustat firm count, N_{\text{Compustat}} , and divide it by the portion of firms that are public, P_{\text{public}} . This gives an estimate of N_{\text{total}} , the total number of firms within the given size bin:
- Next, we test for agreement with the BDS data. We sum our estimated firm counts, N_{\text{total}} , across all bins with 10,000+ employees. We divide this by the equivalent firm count from the BDS, N_{\text{BDS}} . The result is the error ratio \epsilon , which tells us how much we have over/under-estimated the number of US firms with 10,000+ employees:
- Returning to the Compustat database, we now estimate the number of public firms within each firm-size bin. To account for public firms not included in the Compustat database, we take our Compustat firm counts, N_{\text{Compustat}} within each bin, and divide by the error factor \epsilon :
The results of the above calculations are binned firm counts of US public firms. We could then estimate private firm counts, N_{\text{private}} , by subtracting (within each bin) public firm counts from the BDS firm counts:
The problem with doing this directly, however, is that the BDS bins are not ‘nicely’ spaced. (The bins are neither linear nor logarithmic, so using them leads to histograms that are difficult to interpret.) To better represent the data, I transform the BDS firm size bins in the steps below.
Transforming firm-size bins
To transform BDS firm counts N_{\text{BDS}} into bin sizes of my choosing, I use the following procedure:
- Assume that data within each firm-size bin is distributed according to a power law. In the ith bin, the probability of finding a firm with E employees is:
- Initiate all power-law exponents \alpha_i to the best-fit value \alpha for the entire distribution. To estimate \alpha for the US firm size distribution, I follow the binned maximum likelihood method outlined by Virkar and Clausett.
- For the ith bin, choose the constant c_i such that the density function P_i(E) integrates to the BDS firm count N_i over the bin range E_{\text{lwr}} to E_{\text{upr}} :
- At bin edges, E_{\text{edge}} , calculate the difference in the probability density of adjacent bins. That is, take P_i evaluated at E_{\text{edge}} and subtract P_{i+1} evaluated at the same edge E_{\text{edge}} :
- Sum the resulting errors, \epsilon_i , over all adjacent edges. Use the R function
optim
to find the parameters \alpha_i that minimize the sum of \epsilon_i . - With the resulting best-fit values for \alpha_i , simulate the size distribution of firms within each bin. From a discrete power law distribution, select N_i random numbers E , representing the employment of individual firms. Restrict E to be within the bounds of the bin, E_{\text{lwr}} and E_{\text{upr}} :
- Combine the simulated data for each bin, giving a simulation for the size distribution of US firms.
- Get simulated firm counts, N_{\text{sim}} , over log-spaced bins of our choosing.
- Get public firm counts ( N_{\text{public}} , calculated using the steps outlined in the previous section) evaluated over the same log-spaced bins.
- Within each bin, define the number of private firms as the difference between the N_{\text{sim}} and N_{\text{public}} :
This combination of empirical and simulated data gives the results shown in Figure 15. The public portion of US firms roughly follows a log-sigmoid function of firm size.

Notes
- Chaisson’s book Cosmic Evolution is epic, but requires some knowledge of physics. (It contains quite a few equations.) For an equation-free telling of the same story, see Chaisson’s later book Epic of Evolution: Seven Ages of the Cosmos.↩︎
- What is amazing is that equations derived from studying simple mechanical systems seem to apply to the entire universe. In the 17th and 18th century, physicists discovered that gases obey a simple formula. Take pressure P, multiply it by volume V, and divide by temperature T. The result is always constant:
\displaystyle \frac{P \cdot V}{T} = k
This equation is called the ‘ideal gas law’, and it tells us that when a system expands, temperature will decrease. That’s true in your air conditioner. And it’s true for the universe as a whole.↩︎
Further reading
Brenner, R. (1976). Agrarian class structure and economic development in pre-industrial Europe. Past & Present, (70), 30–75.
Carlen, J. (2016). A brief history of entrepreneurship: The pioneers, profiteers, and racketeers who shaped our world. Columbia University Press.
Chaisson, E. (2002). Cosmic evolution: The rise of complexity in nature. Cambridge, Mass.: Harvard University Press.
Chaisson, E. (2005). Epic of evolution: Seven ages of the cosmos. New York: Columbia University Press.
Dunbar, R. I. (1993). Coevolution of neocortical size, group size and language in humans. Behavioral and Brain Sciences, 16(04), 681–694.
Fix, B. (2019). Energy, hierarchy and the origin of inequality. Plos One, 14(4), e0215692.
Frank, A., & Gills, B. (1996). The world system: Five hundred years or five thousand? Psychology Press.
Gould, S. J. (1996). Full house: The spread of excellence from Plato to Darwin. Three Rivers Press.
Hatton, I. A., Dobson, A. P., Storch, D., Galbraith, E. D., & Loreau, M. (2019). Linking scaling laws across eukaryotes. Proceedings of the National Academy of Sciences, 116(43), 21616–21622.
Nitzan, J., & Bichler, S. (2009). Capital as power: A study of order and creorder. New York: Routledge.
Suaste Cherizola, J. (2021). From commodities to assets: Capital as power and the ontology of finance. Review of Capital as Power, 2(1), 1–29.
Virkar, Y., & Clauset, A. (2014). Power-law distributions in binned empirical data. The Annals of Applied Statistics, 8(1), 89–119.
Wallerstein, I. (1974). The modern world-system I: Capitalist agriculture and the origins of the European world-economy in the sixteenth century (1st ed.). Academic Press Inc.
Weststeijn, A. (2014). The VOC as a company-state: Debating seventeenth-century Dutch colonial expansion. Itinerario, 38(1), 13–34.